Data management is a challenge that FinTech (financial technology) companies grapple with due to the consistency in the growth of data volumes. Statista’s findings show that global data consumption is expected to grow to 180 zettabytes in 2025 from 64.2 zettabytes in 2020.
This statistic demonstrates the need for various industries, not only FinTech companies, to embrace data management solutions like machine learning technology to keep up with the data dynamics. The use cases of machine learning in FinTech prove that it is gaining popularity in the industry.
Machine Learning Defined
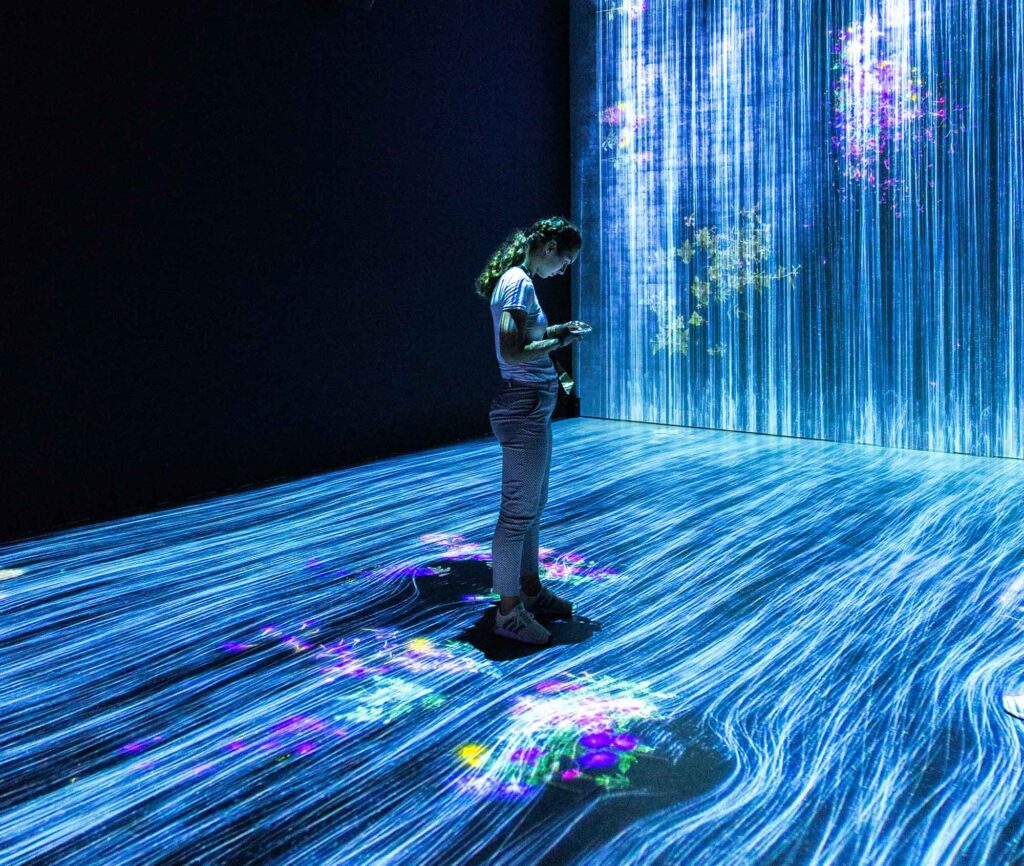
Machine learning is an algorithm-structured element of artificial intelligence that teaches computers data that they can analyze, classify, predict and draw decisions accurately. The use of machine learning is a common phenomenon in the finance sector, where companies and startups use this technology for process automation and to increase efficiency in their daily operations, such as analyzing clients’ creditworthiness.
The self-run technology gets better with time the more it is customed to learn and interpret data patterns. Machine learning is widely used in finance settings such as the stock market, banking, crypto exchange, and insurance. More than ever, FinTech companies are inclined to utilize machine learning to keep up with the increasing industry demands and curb threats like money laundering and other fraudulent activities.
FinTech companies understand that adopting machine learning technology is a sure way of building lasting relationships with their customers because costly issues like fraud have always been a major concern since digitized transactions became a thing. Additionally, the impact of the Corona pandemic has shown why digitization of services is the way to go. There are several ways that FinTech companies can streamline their activities using machine learning.
Ways FinTech Companies Benefit From Machine Learning
FinTech companies usually serve many customers hence the need to find reliable techniques for handling big data. With the adaptation of machine learning, companies can help acquire more customers. Below are ways in which machine learning has proven beneficial for FinTech:
Fraud Detection
Fraud-related cases are a menace that FinTech companies have tried to contain for years. Enter machine learning – and transactions couldn’t be more secure through its fraud-detecting algorithm.
Banks and insurance companies have been on the receiving end of cyber attacks, but investing in machine learning technology has proved rewarding because the computer algorithm has been taught to detect suspicious transactions by cardholders or outsiders based on their transactional behavior or history.
Companies like Paypal have invested in machine learning technology to combat fraud. Machine learning technology can tell apart legal transactions and fraud faster than humans, thus playing a critical role in detection and prevention. FinTechs that use machine learning enjoy customer retention and acquisition because of mitigating fraud significantly.
Forecasting Market Trends
FinTechs can meet their financial goals by relying on machine learning to learn future economic patterns and opportunities and make informed decisions. Through machine learning forecasting, FinTech companies can work with sustainable budgets and save costs.
The technology analyzes records and gives insights that help companies to identify future market patterns, enabling them to make informed investment decisions. Such wouldn’t be possible using traditional models that cannot forecast whether emerging trends are worth investing in. Machine learning is, therefore, reliable in helping companies act swiftly on potentially rewarding investments.
Improved Customer Service
Customer satisfaction is key in the financial sector because it can make or break a company. FinTech companies that know better use machine language to care for their customers’ needs. Machine learning helps financial companies to offer tailored solutions when interacting with customers on various queries.
Through machine learning, FinTech institutions engage their customers and prospects 24/7 through chatbots that are robotic support staff. The traditional way of queuing to get answers on simple matters consumes a lot of time, but machine language can facilitate such in seconds. FinTech companies have given their chatbots human names so that customers can feel comfortable engaging them.
Customers can therefore count on the company’s customer support whenever they need clarification on certain financial-related matters.
Credit Assessment
Given the number of loan applications, most financial companies deal with daily, determining which applicants deserve approval can be hectic if there is no system to help. Machine learning applications have bridged that gap by enabling companies to conduct system checks and award loans to deserving applicants.
Documents can get forged or falsified in the manual loaning process, but machine learning can easily detect such discrepancies and raise the alarm. Machine learning is reliable in identifying potential fraudulent borrowers that cost companies a lot of money.
Large Data Management
Financial customers rely on customer data to run their daily operations, implying they have much to lose if they fail to manage it. Machine language helps financial agencies to collect and compute customer data.
Once the system has been fed with the data, it improves productivity by uncovering patterns and detecting inaccuracies. There is no data limit when using machine language to store or interpret customer data, enabling companies that deal with large volumes of data to streamline their operations.
Simplified Marketing
FinTech companies can use customer data classified through machine learning to strategize effective marketing strategies. Machine learning helps with analytics, a crucial aspect of marketing for companies that want to generate more sales and improve user interface, convincing their customer to stick around.
Among the customer behavioral patterns that machine learning can analyze include purchase patterns, frequently visited web pages, and the time spent, age, gender, etc. The named demographics are marketing essentials that help companies to execute result-oriented marketing campaigns.
Conclusion
Incorporating machine language in the finance sector is a win-win situation for both companies and customers. Customers enjoy smooth services, and companies increase productivity and revenue. However, FinTech companies have more to lose for failing to adopt machine language.
There is no doubt that machine learning is worth investing in because it empowers companies to meet customer demands in a competitive environment. Startups and established FinTech companies should embrace machine learning to reduce operating costs through sustainable technologically-advanced solutions.